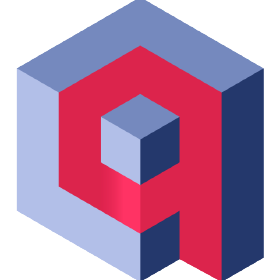
Qdrant Cluster
High-performance Vector Database and Vector Search Engine
Qdrant Peer 2
qdrant/qdrant:v1.12.5
Just deployed
/qdrant/storage
Qdrant Peer 1
qdrant/qdrant:v1.12.5
Just deployed
/qdrant/storage
Qdrant Peer 3
qdrant/qdrant:v1.12.5
Just deployed
/qdrant/storage
Qdrant Main
qdrant/qdrant:v1.12.5
Just deployed
/qdrant/storage
Vector Search Engine for the next generation of AI applications
Qdrant (read: quadrant) is a vector similarity search engine and vector database. It provides a production-ready service with a convenient API to store, search, and manage points—vectors with an additional payload Qdrant is tailored to extended filtering support. It makes it useful for all sorts of neural-network or semantic-based matching, faceted search, and other applications.
Qdrant is written in Rust 🦀, which makes it fast and reliable even under high load. See benchmarks.
With Qdrant, embeddings or neural network encoders can be turned into full-fledged applications for matching, searching, recommending, and much more!
Features
Filtering and Payload
Qdrant can attach any JSON payloads to vectors, allowing for both the storage and filtering of data based on the values in these payloads. Payload supports a wide range of data types and query conditions, including keyword matching, full-text filtering, numerical ranges, geo-locations, and more.
Filtering conditions can be combined in various ways, including should
, must
, and must_not
clauses,
ensuring that you can implement any desired business logic on top of similarity matching.
Hybrid Search with Sparse Vectors
To address the limitations of vector embeddings when searching for specific keywords, Qdrant introduces support for sparse vectors in addition to the regular dense ones.
Sparse vectors can be viewed as an generalization of BM25 or TF-IDF ranking. They enable you to harness the capabilities of transformer-based neural networks to weigh individual tokens effectively.
Vector Quantization and On-Disk Storage
Qdrant provides multiple options to make vector search cheaper and more resource-efficient. Built-in vector quantization reduces RAM usage by up to 97% and dynamically manages the trade-off between search speed and precision.
Distributed Deployment
Qdrant offers comprehensive horizontal scaling support through two key mechanisms:
- Size expansion via sharding and throughput enhancement via replication
- Zero-downtime rolling updates and seamless dynamic scaling of the collections
Highlighted Features
- Query Planning and Payload Indexes - leverages stored payload information to optimize query execution strategy.
- SIMD Hardware Acceleration - utilizes modern CPU x86-x64 and Neon architectures to deliver better performance.
- Async I/O - uses
io_uring
to maximize disk throughput utilization even on a network-attached storage. - Write-Ahead Logging - ensures data persistence with update confirmation, even during power outages.
API
REST
Online OpenAPI 3.0 documentation is available here. OpenAPI makes it easy to generate a client for virtually any framework or programming language.
You can also download raw OpenAPI definitions.
gRPC
For faster production-tier searches, Qdrant also provides a gRPC interface. You can find gRPC documentation here.
Clients
Qdrant offers the following client libraries to help you integrate it into your application stack with ease:
- Official:
- Community:
Where do I go from here?
- Quick Start Guide
- End to End Colab Notebook demo with SentenceBERT and Qdrant
- Detailed Documentation are great starting points
- Step-by-Step Tutorial to create your first neural network project with Qdrant
Template Content
Qdrant Peer 2
qdrant/qdrant:v1.12.5Qdrant Peer 1
qdrant/qdrant:v1.12.5Qdrant Peer 3
qdrant/qdrant:v1.12.5Qdrant Main
qdrant/qdrant:v1.12.5